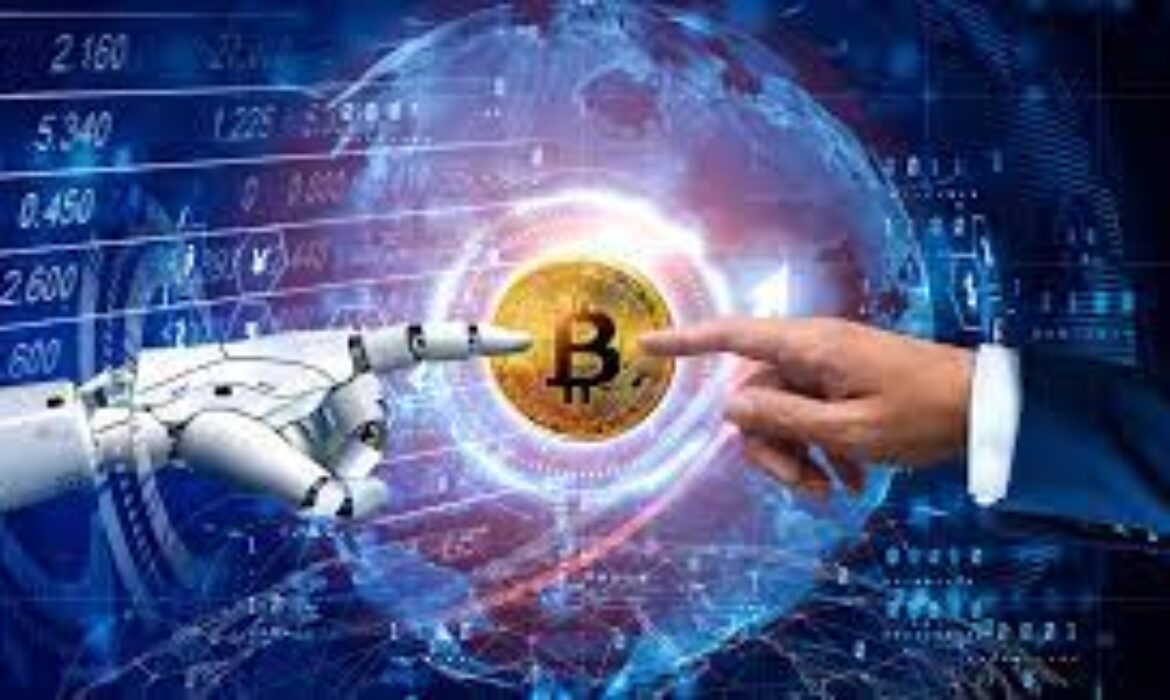
Bayesian networks provide a probabilistic graphical model that represents a set of variables and their conditional dependencies through a directed acyclic graph. They are increasingly applied in risk assessment and decision-making in cryptocurrency trading. Bayesian networks are used to analyze and quantify complex interdependencies among risk factors, combining data with expert judgment to offer rigorous risk quantification and decision support.
A study published in the Journal of Risk and Financial Management examined the statistical properties of major cryptocurrencies, including Bitcoin. It found that Bayesian networks effectively model the complex relationships between various factors affecting cryptocurrency prices. Bayesian networks offer advantages in capturing these interdependencies, providing a structured way to assess risks and predict outcomes using historical data and observed trends.
Another study in the ISACA Journal highlighted the capability of Bayesian networks in cyber risk assessment, demonstrating their ability to capture intricate interdependencies among risk factors and offer a comprehensive understanding of risk. These models are adaptable to the context of cryptocurrency trading, where they can handle the intricate and volatile nature of the market.
Data Sources and Integration
In crypto trading, researchers use datasets from cryptocurrency exchanges, aggregated cryptocurrency indexes, and user forums. These datasets typically include price, trading volume, order-level information, and sentiment-based data with emotional or statistical labels. The data resolution or latency of these datasets is crucial to distinguish between high-frequency and low-frequency trading strategies.
Bayesian networks in sentiment analysis for cryptocurrency trading leverage natural language processing technology to analyze news and media contents. This approach aims to improve trading strategy performance by incorporating various data sources while updating baseline natural language processing models and applying neural networks in label training. Similar methods have been applied in other sectors, such as health economics and outcomes research. For example, a study in Environmental Health applied Bayesian networks to quantify the association between lead exposure and amyotrophic lateral sclerosis, and another study in the Journal of the American Heart Association used them to predict coronary heart disease risk from electronic health records.
Predictive Capabilities and Hybrid Models
The predictive capabilities of Bayesian networks are demonstrated in various applications, including activity pattern decisions and transportation models. Studies have shown that Bayesian networks outperform traditional decision tree models, capturing behavioral mechanisms and predicting outcomes more effectively. This predictive power is particularly relevant to crypto trading, where market behavior is influenced by multiple factors and can be unpredictable.
In the realm of hybrid models, combining Bayesian networks with deep learning techniques has shown promise in predicting cryptocurrency prices. A study employing a hybrid model combining long short-term memory (LSTM) networks with attention mechanisms and gradient-specific optimization demonstrated enhanced predictive accuracy for Bitcoin prices. This integration of probabilistic reasoning and machine learning techniques indicates a robust framework for risk assessment and decision-making in crypto trading.
Insights from other sectors further highlight the potential of Bayesian networks. For instance, a dynamic Bayesian network-based risk assessment framework developed for maritime trade routes underscores the importance of adaptability and real-time data integration in managing uncertainties. A study on port entry risk assessment also emphasizes the adaptability and effectiveness of Bayesian models in evaluating decision-making elements and risk assessment in various contexts. These methodologies can be applied to crypto trading to better understand market dynamics and potential risks.
Applications and Future Directions
Bayesian networks have also been applied successfully in other areas, indicating their versatility and broad applicability. For instance, in cyber risk assessment for maritime trade and evaluating decision-making elements, they reveal several parallels applicable to cryptocurrency trading.
A study on the Russian financial market revealed significant network spillover effects between crypto assets and other financial instruments such as equities, exchange rates, and commodities. This interconnectedness implies that crypto assets are crucial in transmitting risk within the financial landscape, an important factor to consider in risk assessment models. Bayesian networks could capture these intricate relationships, providing a comprehensive risk assessment framework for crypto trading.
Incorporating real-time data can further enhance the decision-making process in crypto trading. For example, the digitalization of micro, small, and medium enterprises (MSMEs) in India during the COVID-19 pandemic illustrates how technology can enhance operational efficiency and risk management. These examples underscore the potential for Bayesian networks to adapt to changing market conditions and incorporate real-time data, providing a more agile and responsive approach to risk assessment in crypto trading.
The book “Risk Assessment and Decision Analysis with Bayesian Networks” provides a comprehensive guide to building and using Bayesian network models for practical problem-solving. It includes extensive example models of real-world risk assessment problems in various domains, including finance and safety. This resource underscores the practical utility of Bayesian networks, reinforcing their application in various fields, such as crypto trading.
Researchers also utilize advanced methods like natural language processing and neural networks for crypto trading tools enhancement. This approach improves the performance of trading strategies by integrating video sources and updating baseline natural language processing models. Sentinel analysis, another application area, demonstrates the versatility of Bayesian networks in integrating various data points for comprehensive analysis and prediction.
Studies have demonstrated the applicability of Bayesian networks across different domains, including cryptocurrency trading. The modeling of complex relationships and the predictive power provided by Bayesian networks make them a valuable tool for risk assessment and decision-making in this field. Hybrid models that combine Bayesian networks with machine learning techniques offer additional robustness, making them an attractive option for traders seeking to navigate the uncertainties of cryptocurrency markets effectively.