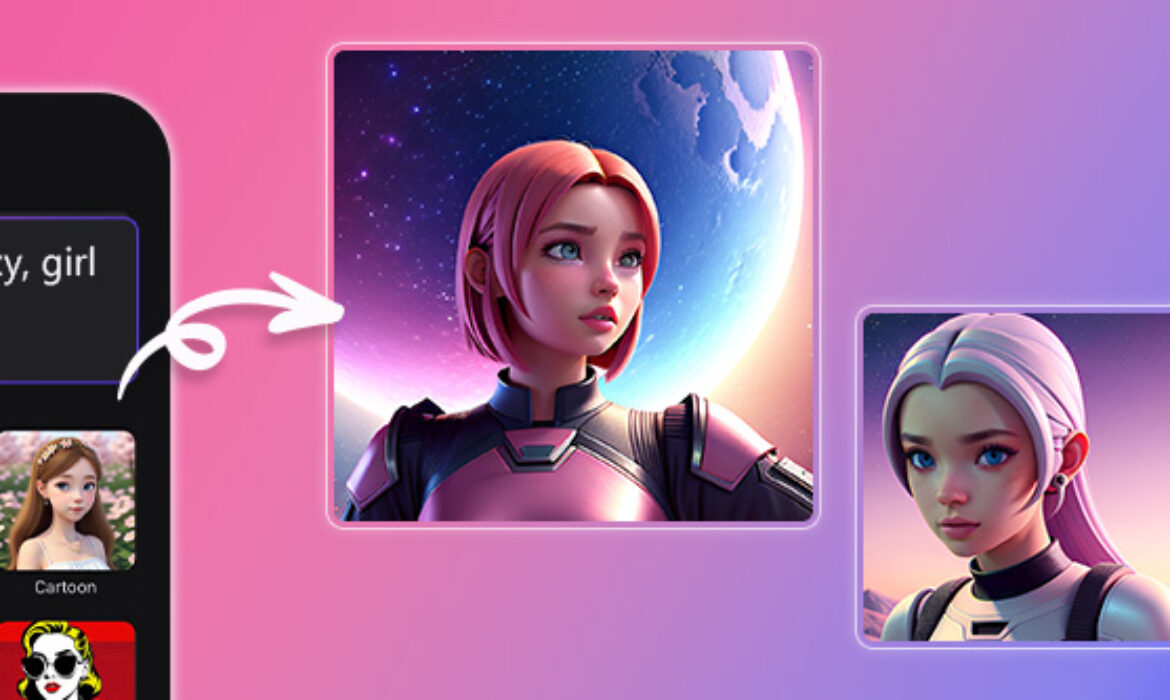
For digital artists, developing striking images from text has become a quite handy and effective weapon. As artificial intelligence develops, it becomes simpler to turn written descriptions into amazing graphics. This book seeks to lead you through the process of creating excellent graphics from text cues, providing useful advice and techniques to increase the potency of your work. Learning the technique of creating exact prompts and using appropriate tools can open fresh creative opportunities and improve your visual material.
Understanding Text-to-Image Technology
Basics of AI and Machine Learning in Image Generation
By allowing computers to produce images from textual descriptions, artificial intelligence (AI) and machine learning (ML) have transformed the discipline of picture production. The capacity of artificial intelligence models to learn from vast collections of images and words forms the core of this technology. Analyzing these sets, machine learning techniques identify trends, characteristics, and correlations between verbal descriptions and visual components. By means of this learning process, the artificial intelligence generates visuals corresponding with the provided text cues, thereby bridging written words with visual representation.
Key Technologies Used: GANs, VAEs, and Diffusion Models
Several advanced technologies drive the capabilities of text-to-image systems:
- Generative adversarial networks (GANs) are: Two neural networks—the generator and the discriminator—that cooperate in a competitive process form GANs. The generator generates pictures from scratch; the discriminator assesses their authenticity. As the generator learns from the feedback of the discriminator, this adversarial training helps it create ever lifelike pictures.
- Variational autoencoders (VAEs) create pictures by use of an encoder-decoder system. The encoder compresses input data into a latent space; the decoder then rebuilds it into a picture. Through learning a structured representation of the data, this method lets VAEs generate varied and coherent images.
- Diffusion Models: Diffusion models create images through a process of gradually refining random noise into structured visuals. These models start with noisy inputs and iteratively denoise them to produce high-quality images. The ability to generate detailed and realistic visuals stems from the model’s training to reverse the noise process.
Overview of How Text Prompts Are Converted into Images
The conversion of text prompts into images involves several steps. First, the AI system interprets the text prompt using natural language processing (NLP) techniques to understand the desired visual elements. The interpreted prompt is then fed into the chosen image generation model—be it a GAN, VAE, or diffusion model. The model processes the input to generate an image that reflects the description provided in the text. Throughout this process, the AI applies its learned knowledge to translate textual features into visual components, resulting in a final image that aligns with the prompt’s details and context.
Preparing Your Text Prompts
Crafting Clear and Detailed Prompts
- Value of particularity Achieving the greatest outcomes from text-to– picture generators depends on well crafted, specific cues. By helping the artificial intelligence to precisely know what you want to produce, specificity lowers uncertainty and raises the accuracy of the produced images. Clear directions on the elements, style, and context of the image from a well-defined prompt help to produce images that quite fit your expectations.
- Examples of Effective vs. Ineffective Prompts Effective prompts are those that include specific details and context. For instance, “A serene landscape with a calm lake, surrounded by snow-capped mountains under a clear blue sky” is more likely to produce a satisfying image than a vague prompt like “A beautiful scene.” Ineffective prompts lack detail and can result in generic or irrelevant images. By including descriptive elements and context, you can guide the AI more precisely and obtain better visual outcomes.
Utilizing Descriptive Language
- Adding Context and Details To create images that align with your vision, it is essential to use descriptive language that adds context and detail to your prompts. This includes specifying the setting, mood, colors, and any other relevant aspects that contribute to the overall image. For instance, “A bustling city street at sunset, with colorful neon signs and a lively crowd” offers a richer picture than just “A city street,” thereby enabling the artificial intelligence to create a more realistic and interesting image.
- Using vivid descriptions and adjectives Strong descriptions and adjectives help to improve the question by expressing the intended visual traits and feelings. Words like “vibrant,” “majestic,” “gloomy,” or “rustic” provide the AI further layers of detail that direct it in producing visuals that arouse particular emotions or traits. For instance, “A majestic old oak tree with spreading branches and a dense, leafy canopy” is more likely to produce a visually appealing outcome than “A tree.”
Testing and Refining Prompts
- Iterative Approach: Adjusting Prompts Based on Results The process of generating images from text prompts often involves testing and refining your prompts. Start with an initial description and evaluate the generated images. Based on the results, make adjustments to your prompts to better align with your vision. This iterative approach allows you to fine-tune the prompts, enhancing the accuracy and quality of the images over time.
- Common Pitfalls to Avoid When crafting prompts, avoid common pitfalls such as being too vague or using contradictory terms. Ambiguity can lead to unsatisfactory results, as the AI may struggle to interpret your intent. Additionally, ensure that your prompts are internally consistent and clear, avoiding descriptions that might confuse the AI or lead to conflicting visual elements. By addressing these issues, you can improve the effectiveness of your prompts and achieve better image generation outcomes.
Conclusion
Mastering the art of creating stunning images from text prompts unlocks a realm of creative possibilities, allowing you to transform written descriptions into vibrant visuals. By understanding the technology behind text-to-image generation and preparing well-crafted, detailed prompts, you can guide AI tools to produce images that align closely with your vision. The process involves specifying details, using descriptive language, and refining prompts through iterative adjustments. Embracing these practices not only enhances the quality of your generated images but also expands your creative potential.
Read MoreFrom C-incognito